The data science ecosystem encompasses data fairness, statistical, ML methods and tools, interpretable data analysis, and trustworthy decision-making. Rapid advancements in ML have revolutionized data utilization and enabled machines to learn from data more effectively. Statistics, as the science of learning from data while accounting for uncertainty, plays a pivotal role in addressing complex real-world problems and facilitating trustworthy decision-making. In this talk, I will discuss the challenges and opportunities involved in building an end-to-end scalable and interpretable data science ecosystem that integrates statistics, ML, and domain science. I will illustrate key points using the analysis of whole genome sequencing data and electronic health records by discussing a few scalable and interpretable statistical and ML methods, tools and data science resources, using large annotation databases, summary statistics, sparsity, and ensemble methods. This talk aims to ignite proactive and thought-provoking discussions, foster collaboration, and cultivate open-minded approaches to advance scientific discovery.
嘉宾介绍
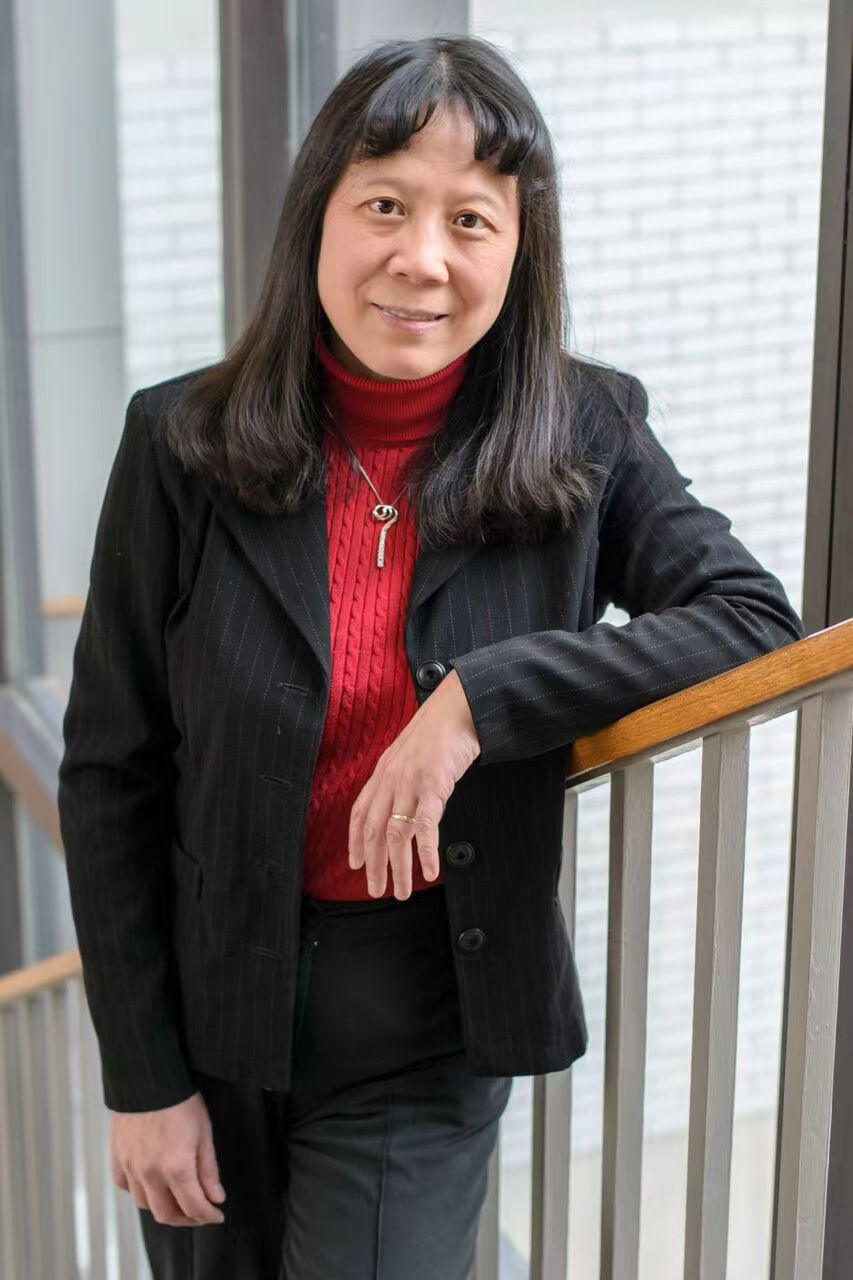
林希虹
美国国家科学院院士